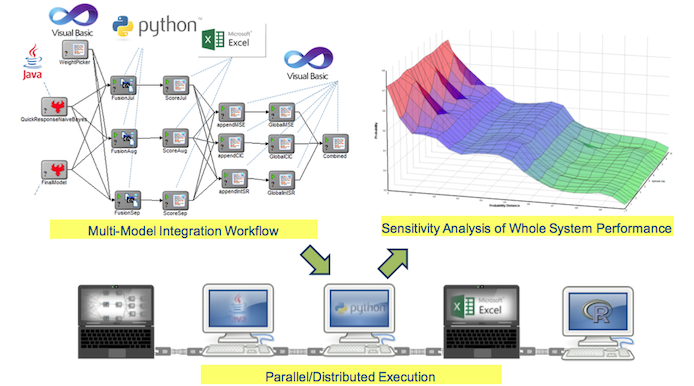
George Mason University is supporting Innovative Decisions, Inc. on a team sponsored by IARPA to study inference enterprise modeling. An inference enterprise consists of data, tools, people and processes employed by an organization to make evidence-based inferences. An inference enterprise model uses available information about an inference enterprise to predict performance of the enterprise. This is a challenging task because data are often incomplete and noisy, and may be aggregated to protect privacy. For this reason, models may require judgmental inputs from experts as well as sophisticated approaches to addressing incomplete data. Our multi-modeling approach to Inference Enterprise Modeling (IEM), or MIEM, constructs multiple models to generate multiple predictions of IEM performance, which are combined into an overall estimate of performance with error bounds. In keeping with well-established results on ensemble models, the MIEM approach provides more accurate predictions than the individual models.
Combining different models that have not been designed to work together, and may use different data or depend on different assumptions, is a major integration challenge. We have developed a methodology and supporting tools to enable modelers to automate complex modeling workflows, focusing on performing complex analyses and communicating results, without being consumed with integration details. Our multi-modeling platform provides facilities for sensitivity analysis of model parameters to identify key drivers of whole system performance. Our Semantic Testbed for Inference Enterprise Modeling (STIEM) allows construction of modeling workflow that can be instantiated with multiple data sources and different parameter values of the detection algorithms. The workflow is automated for simulating the given enterprise model.
We have demonstrated an application of MIEM to the insider threat domain, specifically to estimating how well system alerts based on insider behaviors perform at identifying insiders of true concern. These alerts are commonly generated via a fusion algorithm and associated threshold(s) from detector data. MIEM connects detector data with knowledge about the population of insiders who behave in ways that could cause concern. The modeling workflows are instantiated in STIEM to simulate an inference enterprise and perform analyses of its performance under different scenarios and assumptions. Also as part of our insider threat modeling effort, our research team has created an advanced insider threat indicator ontology to inform the models.
MIEM is applicable to diverse domains that involve human behavior, detectors, fusion algorithms, threshold settings and missing data on the “threat” behaviors – examples include airport security, event security, anti-terrorism, fraud prevention, and insider trading. MIEM should be particularly useful to organizations that are considering investments in additional detectors or additional processing power, or evaluating new algorithms and thresholds for identifying insiders of concern for further investigation.